Welcome
to the AI era
ofadvertising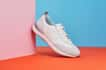


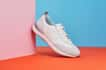

In-depth analysis — intelligent audience targeting — bid and budget optimization — dynamic campaign adjustments — AI-driven recommendations — In-depth analysis — intelligent audience targeting — bid and budget optimization — dynamic campaign adjustments — AI-driven recommendations — In-depth analysis — intelligent audience targeting — bid and budget optimization — dynamic campaign adjustments — AI-driven recommendations — In-depth analysis — intelligent audience targeting — bid and budget optimization — dynamic campaign adjustments — AI-driven recommendations —
trend analysis — intelligent audience targeting — ad budget optimization — dynamic campaign adjustments — AI-driven recommendations — trend analysis — intelligent audience targeting — ad budget optimization — dynamic campaign adjustments — AI-driven recommendations — trend analysis — intelligent audience targeting — ad budget optimization — dynamic campaign adjustments — AI-driven recommendations — trend analysis — intelligent audience targeting — ad budget optimization — dynamic campaign adjustments — AI-driven recommendations —
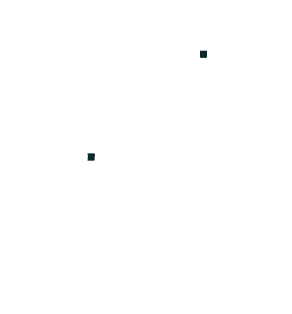

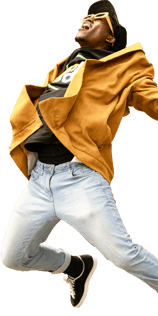
brand-safe creative — instant high-impact visuals — studio-quality ads — creative — feed generator — static to video — cross-platform outputs — bulk asset creation — brand-safe creative — instant high-impact visuals — studio-quality ads — creative — feed generator — static to video — cross-platform outputs — bulk asset creation — brand-safe creative — instant high-impact visuals — studio-quality ads — creative — feed generator — static to video — cross-platform outputs — bulk asset creation — brand-safe creative — instant high-impact visuals — studio-quality ads — creative — feed generator — static to video — cross-platform outputs — bulk asset creation —
brand-safe creative — instant high-impact visuals — studio-quality ads — creative — feed generator — static to video — cross-platform ready — brand-safe creative — instant high-impact visuals — studio-quality ads — creative — feed generator — static to video — cross-platform ready — brand-safe creative — instant high-impact visuals — studio-quality ads — creative — feed generator — static to video — cross-platform ready — brand-safe creative — instant high-impact visuals — studio-quality ads — creative — feed generator — static to video — cross-platform ready —
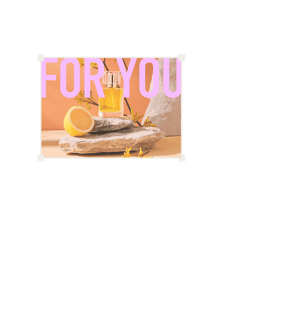

In-depth analysis — intelligent audience targeting — bid and budget optimization — dynamic campaign adjustments — AI-driven recommendations — In-depth analysis — intelligent audience targeting — bid and budget optimization — dynamic campaign adjustments — AI-driven recommendations — In-depth analysis — intelligent audience targeting — bid and budget optimization — dynamic campaign adjustments — AI-driven recommendations — In-depth analysis — intelligent audience targeting — bid and budget optimization — dynamic campaign adjustments — AI-driven recommendations —
trend analysis — intelligent audience targeting — ad budget optimization — dynamic campaign adjustments — AI-driven recommendations — trend analysis — intelligent audience targeting — ad budget optimization — dynamic campaign adjustments — AI-driven recommendations — trend analysis — intelligent audience targeting — ad budget optimization — dynamic campaign adjustments — AI-driven recommendations — trend analysis — intelligent audience targeting — ad budget optimization — dynamic campaign adjustments — AI-driven recommendations —
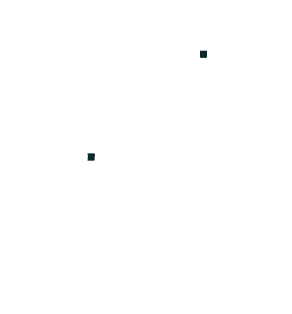

brand-safe creative — instant high-impact visuals — studio-quality ads — creative — feed generator — static to video — cross-platform outputs — bulk asset creation — brand-safe creative — instant high-impact visuals — studio-quality ads — creative — feed generator — static to video — cross-platform outputs — bulk asset creation — brand-safe creative — instant high-impact visuals — studio-quality ads — creative — feed generator — static to video — cross-platform outputs — bulk asset creation — brand-safe creative — instant high-impact visuals — studio-quality ads — creative — feed generator — static to video — cross-platform outputs — bulk asset creation —
brand-safe creative — instant high-impact visuals — studio-quality ads — creative — feed generator — static to video — cross-platform ready — brand-safe creative — instant high-impact visuals — studio-quality ads — creative — feed generator — static to video — cross-platform ready — brand-safe creative — instant high-impact visuals — studio-quality ads — creative — feed generator — static to video — cross-platform ready — brand-safe creative — instant high-impact visuals — studio-quality ads — creative — feed generator — static to video — cross-platform ready —
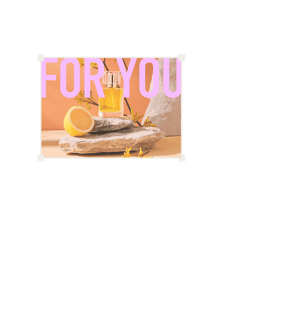
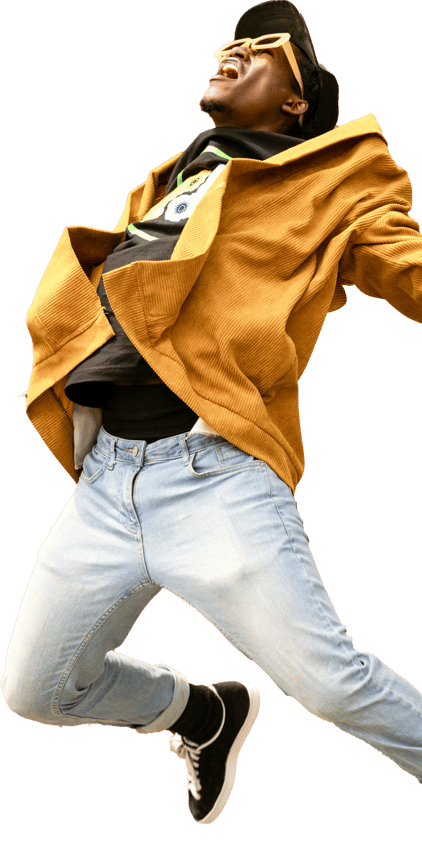
More wins, less waste
More breakthroughs
Jaw-dropping creative, on demand
Powering
adperformanceforglobalbrands


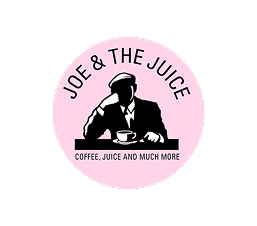
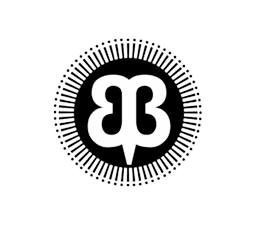
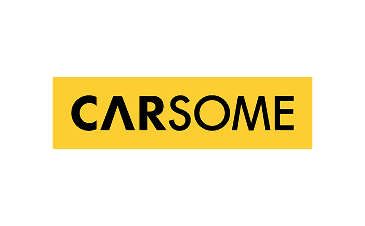
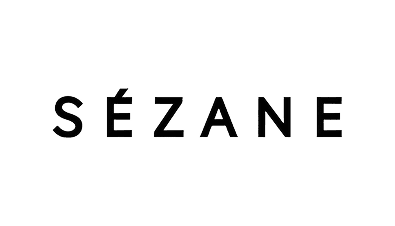
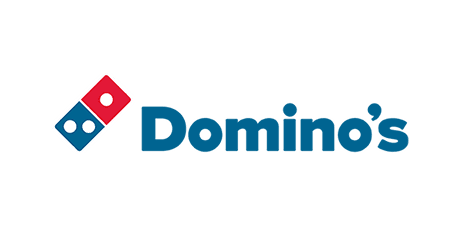
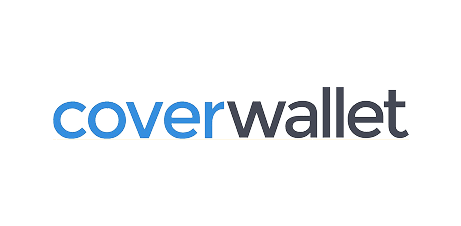
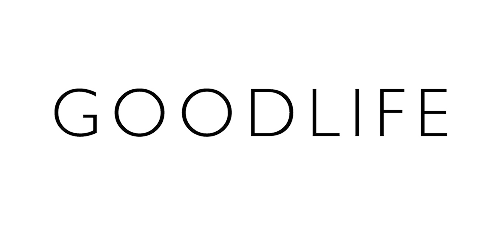
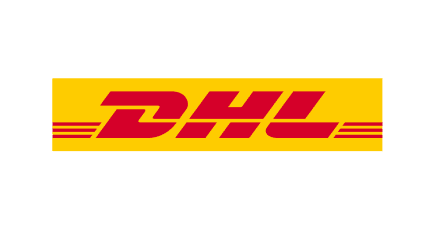
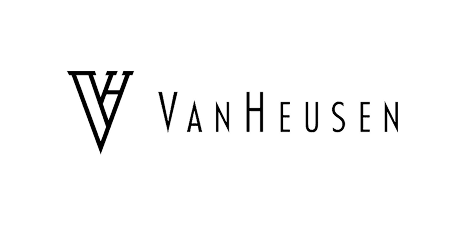
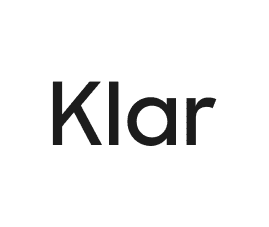
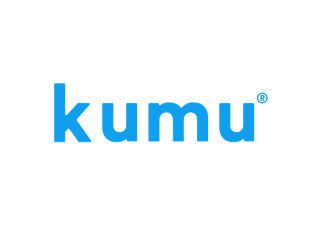
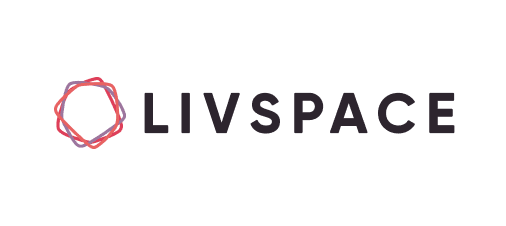
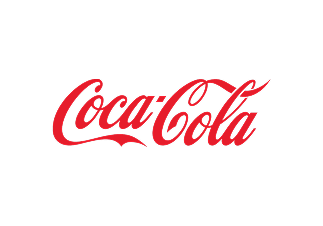
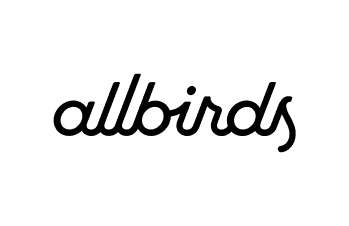
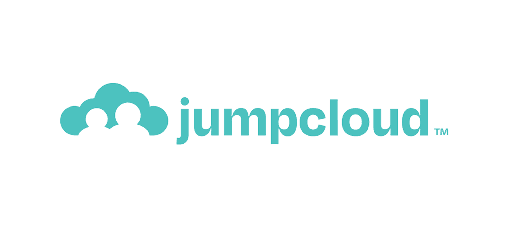
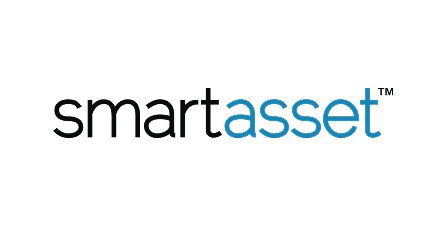
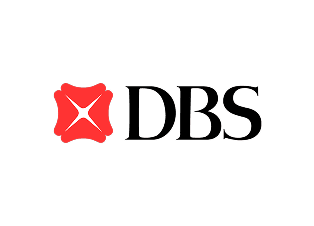
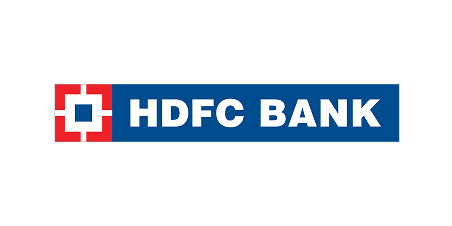
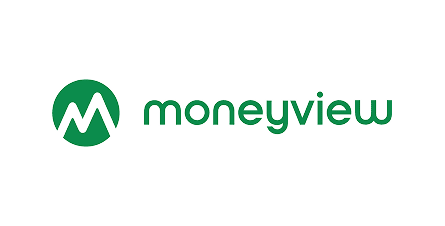
Betabrand Improves Return on Ad Spend (RoAS) by 69%
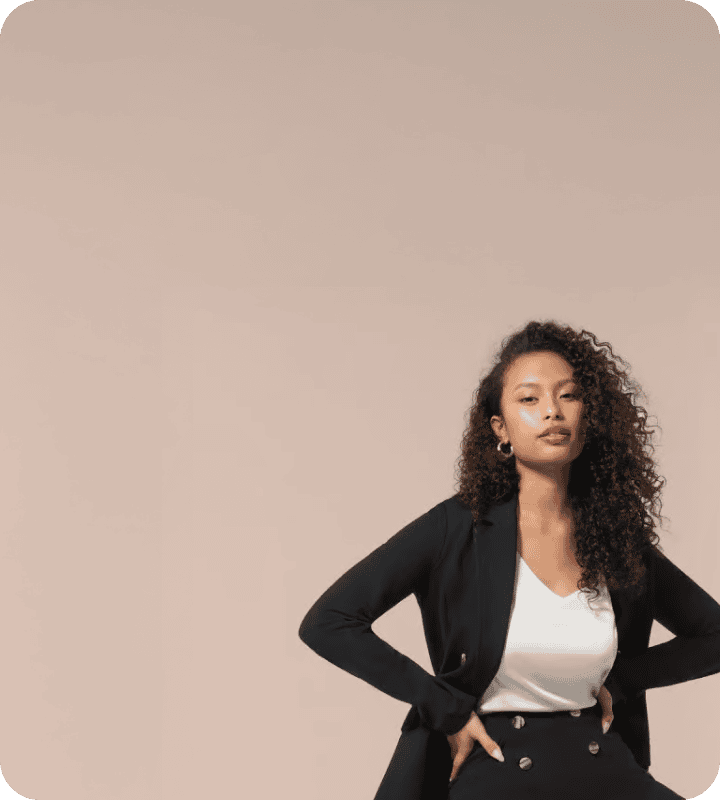
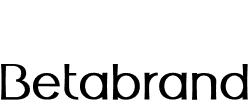
immi Decreases Cross-Channel CPA by 7% While Doubling Ad Spend
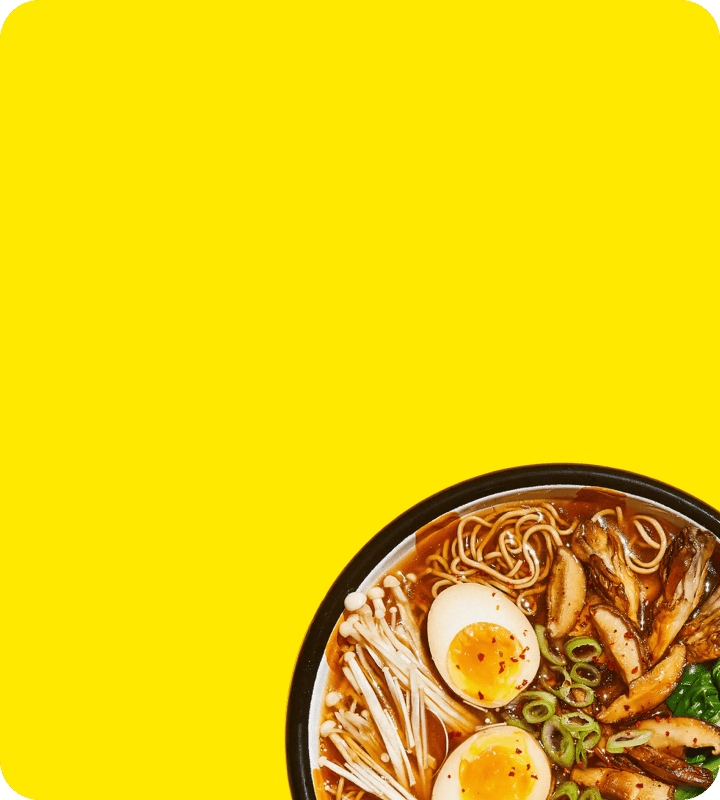
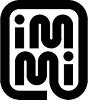
Joe & The Juice Improves its Conversion Rate (CVR) by 14%
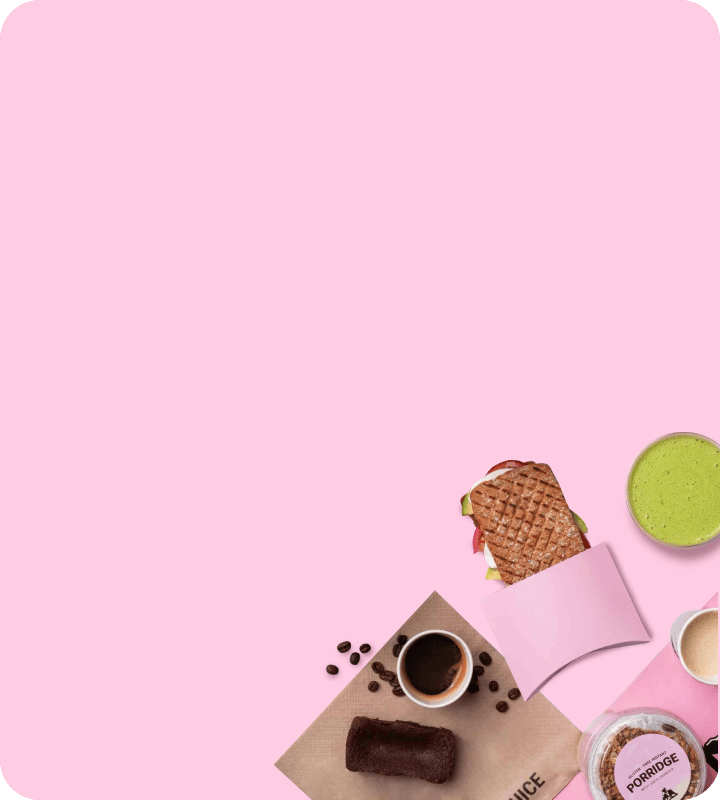
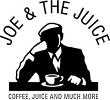
Nomad Reduces CAC by 28% with Pixis
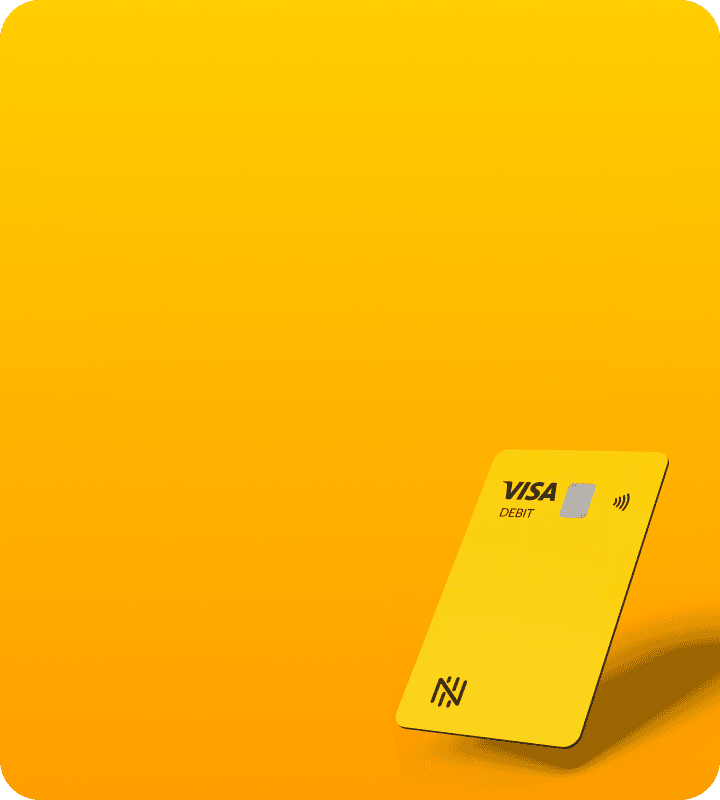
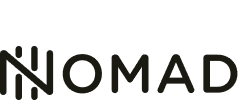
Swiggy reduces Cost per Install by 41%
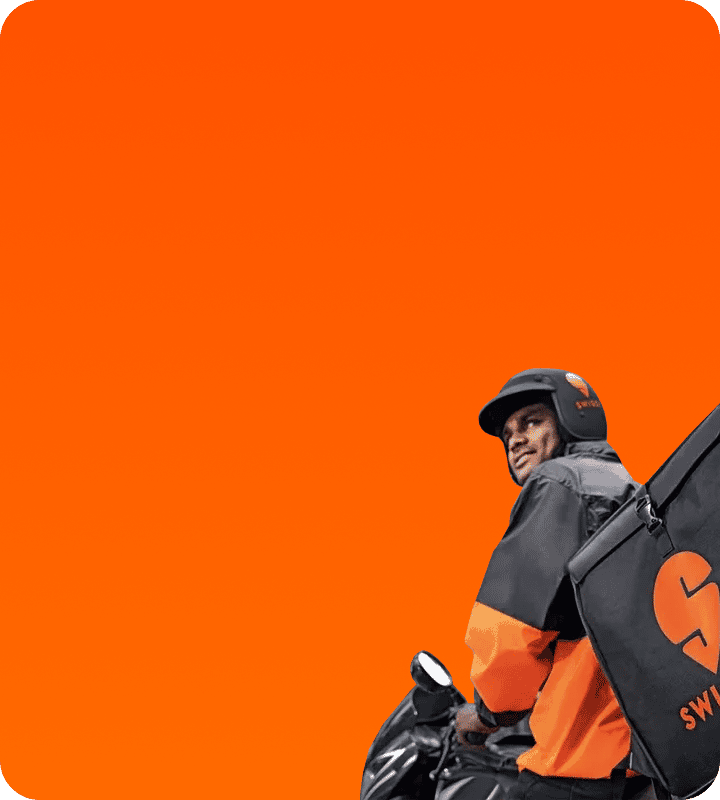
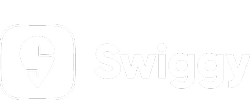
Klar Reduced its Cost Per First Transaction (CPFT) by 29%
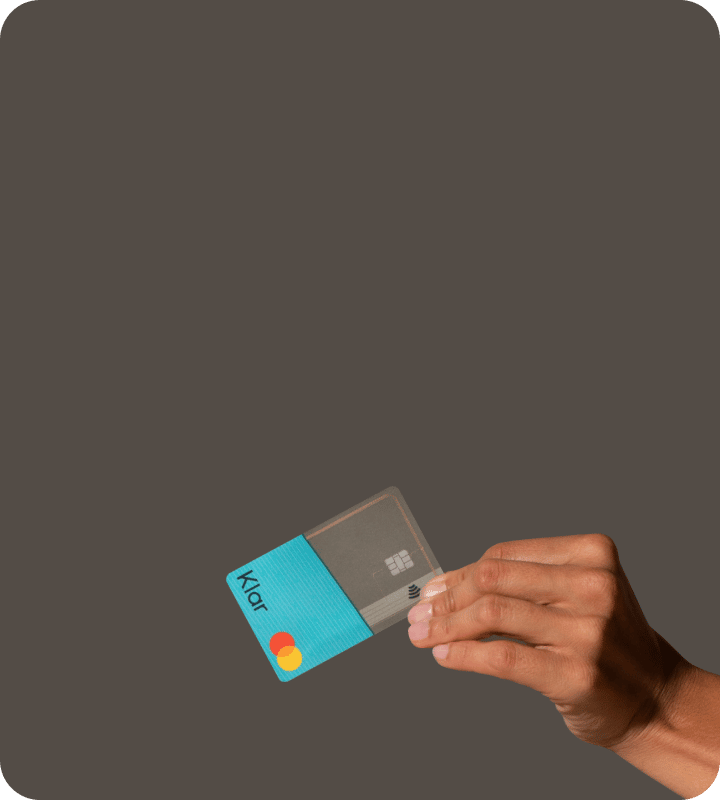
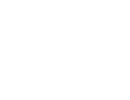
Betabrand Improves Return on Ad Spend (RoAS) by 69%
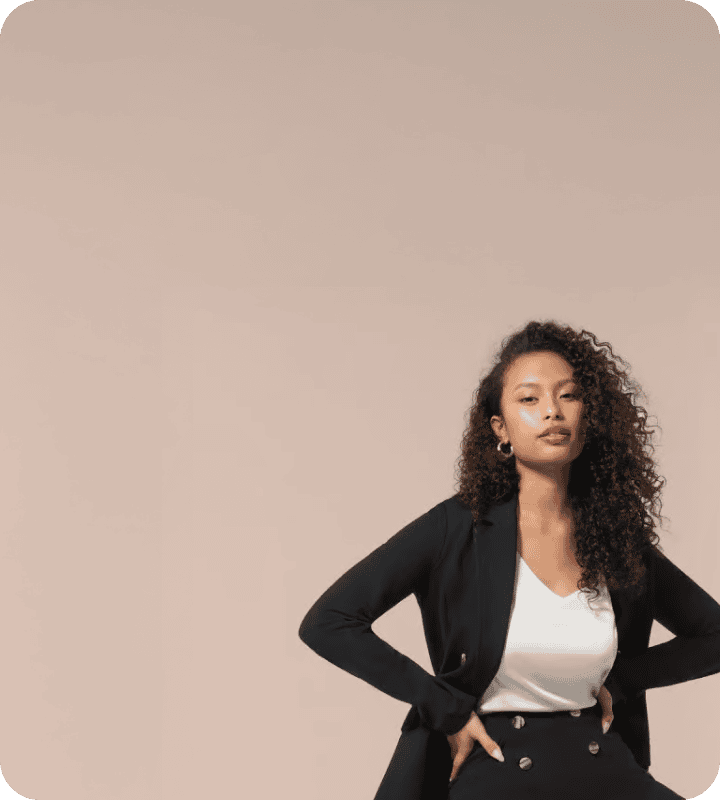
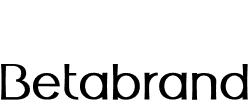
immi Decreases Cross-Channel CPA by 7% While Doubling Ad Spend
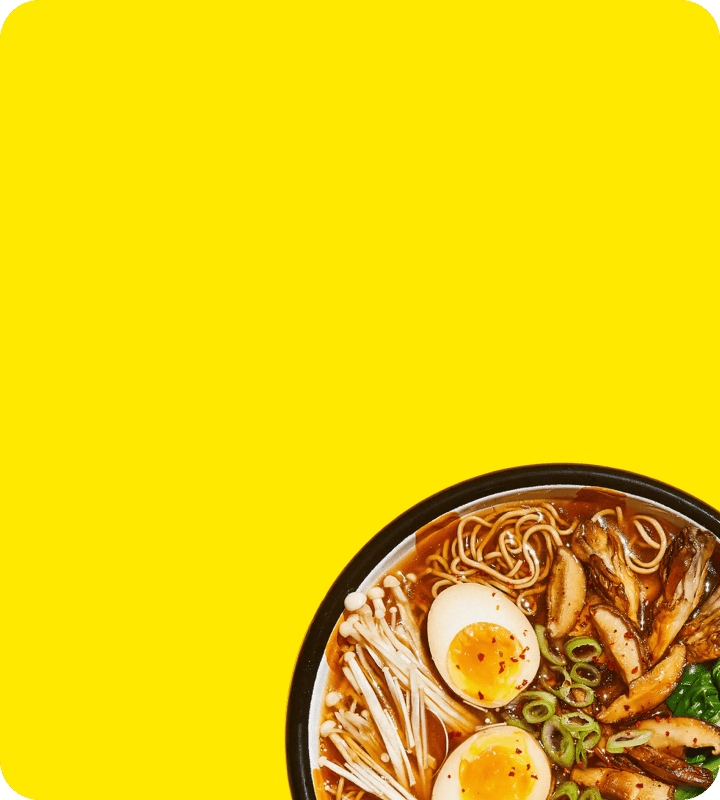
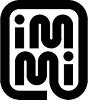
Joe & The Juice Improves its Conversion Rate (CVR) by 14%
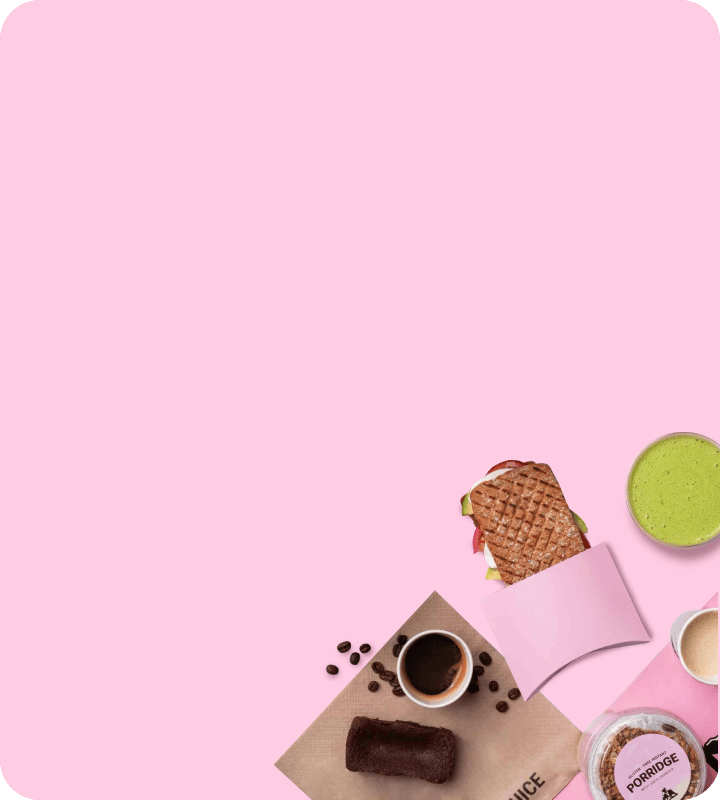
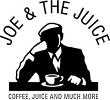
Nomad Reduces CAC by 28% with Pixis
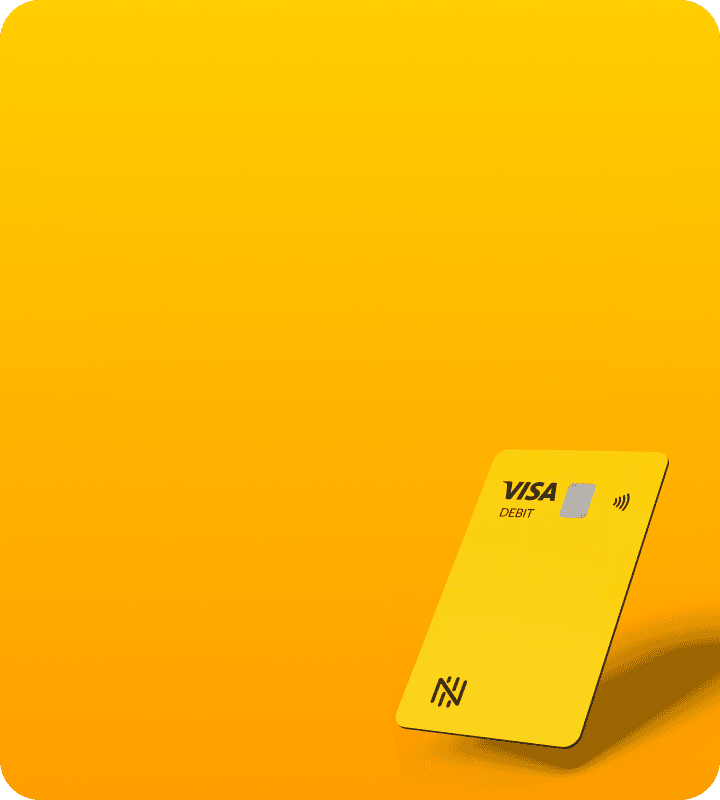
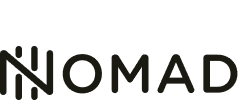
Swiggy reduces Cost per Install by 41%
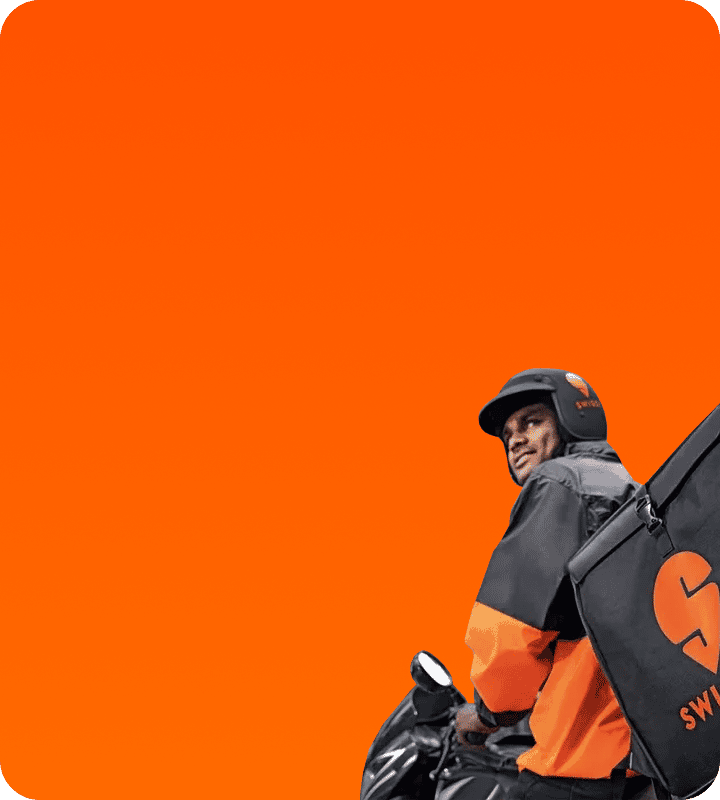
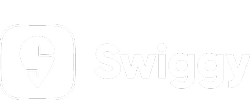
Klar Reduced its Cost Per First Transaction (CPFT) by 29%
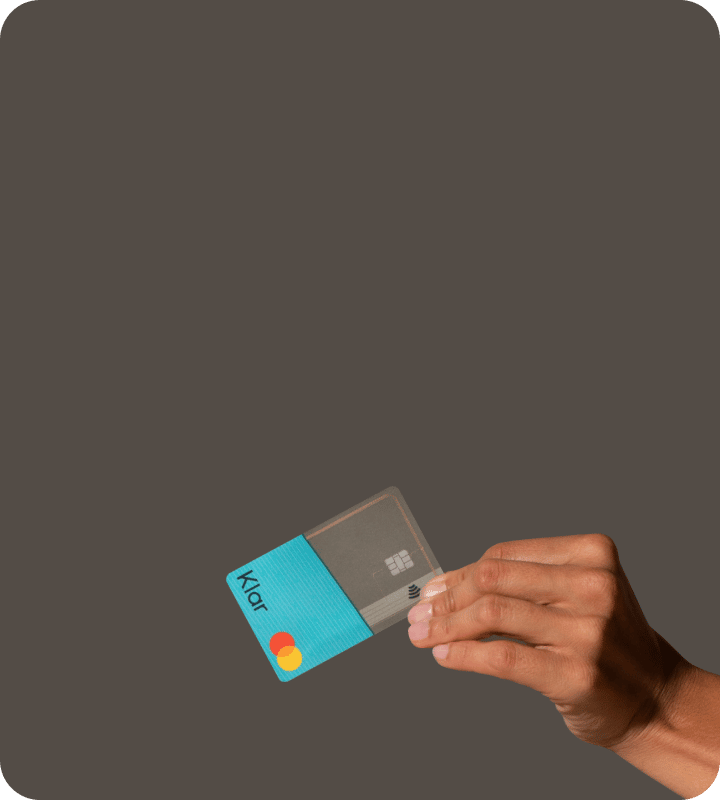
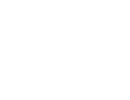