Synthetic vs First-Party Data: Breaking Down the Differences
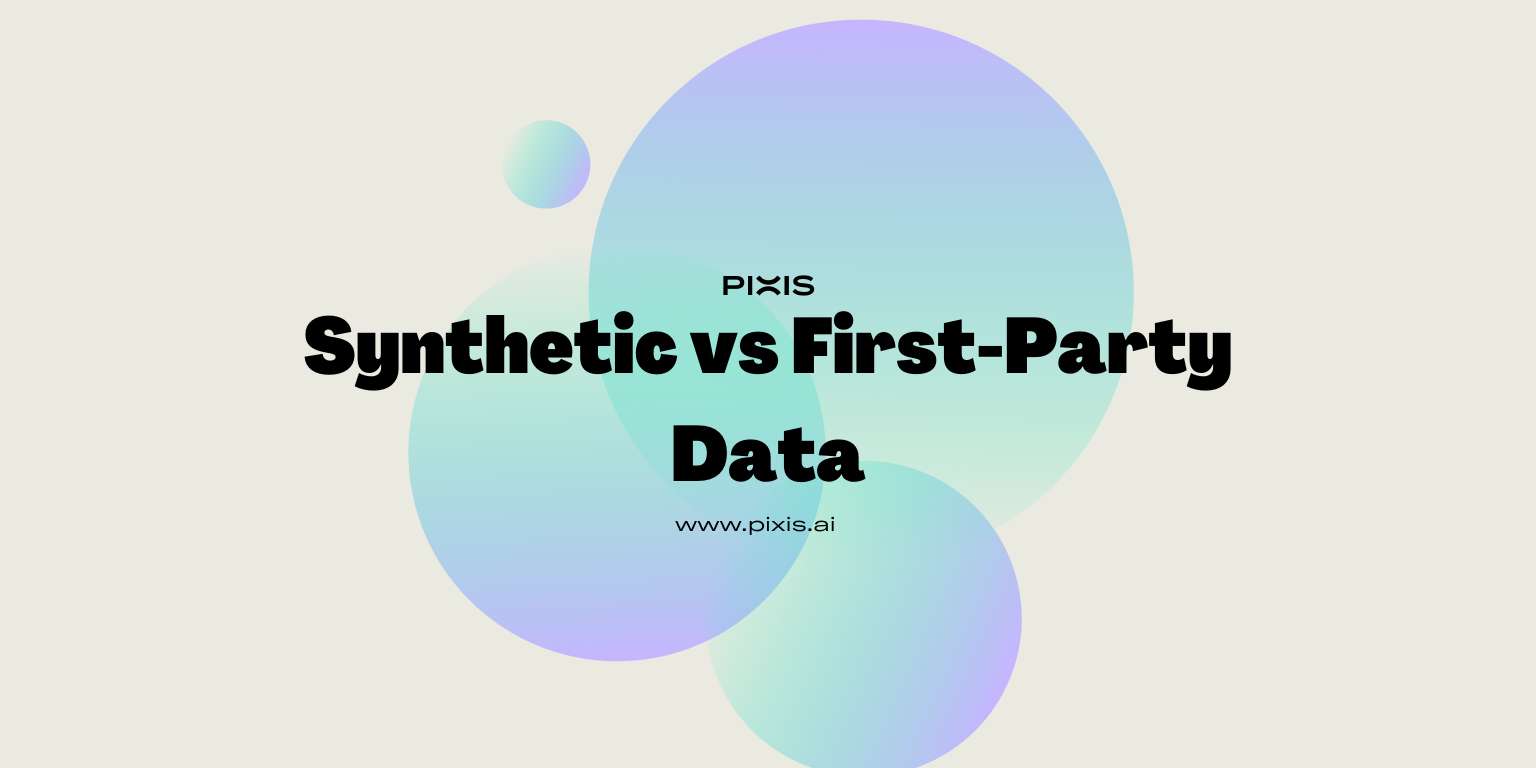
A smart data strategy is integral for B2C marketers with the rising privacy concerns around data. First-party data, gathered directly from customer interactions, is accurate, consent-based, and ideal for personalization, segmentation, and targeting. It reflects real user behavior and builds long-term trust.
Synthetic data, generated algorithmically to mirror real-world patterns, doesn’t rely on actual customer information. This makes it valuable for testing, experimentation, and AI training without privacy risk. While it lacks real-user context, it offers scalability and safety in data-restricted environments.
Each data type serves a different purpose. In the sections below, we’ll break down the key differences, benefits, and use cases to help you decide how to incorporate both into your performance marketing strategy.
What Is First-Party Data?
First-party data is information your business collects directly from your audience through your owned channels and platforms.
Unlike third-party data from external sources, this information comes straight from your customers' interactions with your business.
Common examples of first-party data include website browsing behavior and clicks, purchase history from your store, email engagement metrics, mobile app usage, customer feedback and survey responses, social media interactions with your brand, and subscription information.
What makes first-party data so valuable is its accuracy and relevance to your specific business. Since you're collecting it directly, you know exactly where it came from and how it was gathered. This has become especially important in the wake of privacy changes like Apple’s iOS 14 update, which significantly limited access to third-party tracking data. For consumer brands relying heavily on platforms like Facebook and Instagram for advertising, first-party data now plays a central role in optimizing performance, retargeting, and personalization.
From a privacy standpoint, first-party data gives you a significant advantage when it comes to handling personal information. Since customers willingly provide this information through direct interactions with your brand, you can typically collect it with proper consent, making compliance with regulations like GDPR and CCPA more straightforward.
What Is Synthetic Data?
Synthetic data is artificially generated information that mimics real-world data without containing any actual personal or sensitive information. Rather than being "fake" data, it's algorithmically created to maintain the statistical properties, patterns, and relationships found in authentic datasets.
This data type is typically created through sophisticated computational methods, including machine learning models, statistical distributions, or rule-based systems. For example, algorithms can analyze real customer behavior patterns and generate similar synthetic profiles that preserve significant trends while removing personally identifiable information.
Synthetic data serves multiple central functions in your marketing toolkit. It helps you comply with privacy regulations while still allowing for powerful analytics. You can use it to supplement limited first-party data for more robust modeling, simulate diverse customer scenarios, and test marketing strategies without risk.
Understanding generative AI techniques is important to constructively creating synthetic data that benefits your marketing strategies. You can also develop personalized experiences and predictive models without compromising consumer privacy, an increasingly valuable capability in today's privacy-conscious environment.
What Each Data Type Does Best
Let's examine what synthetic data and first-party data each bring to the table.
1st Party Data Advantages
First-party data, as information collected directly from your customers through your owned channels, offers several distinct advantages:
- Real customers, real insights: Since first-party data comes directly from your customers, it provides authentic insights into their behaviors, preferences, and needs, helping you understand consumer behavior.
- Hyper-personalization made easy: With first-party data, you can create highly personalized experiences based on customer behaviors and preferences. To do this, segment your audience based on their interactions with your brand, then tailor your messaging to their specific interests and needs.
You can also leverage first-party data to create lookalike audiences and expand your reach. Brands like Google and Meta rely heavily on lookalike audiences from first-party data. - Privacy-first and brand-safe: Because customers provide this data directly to you, it's more compliant with privacy regulations like GDPR and CCPA. This direct relationship also builds trust, as customers understand who has their data and how it's being used.
Synthetic Data Advantages
Synthetic data, as an artificially generated information that mimics real data patterns without containing actual personal information, offers its own set of benefits:
- Fast, scalable, and safe: You can generate synthetic data quickly and in large volumes without privacy concerns. This makes it particularly valuable when you need to scale your data operations rapidly while maintaining privacy compliance. A performance marketing team might use a synthetic data generation tool like Gretel.ai to simulate user journeys through a checkout funnel, testing how different demographics or behaviors might respond to a new landing page layout before launching it to a live audience.
- Ideal for model training and testing: Synthetic data provides a robust foundation for training and testing AI models without risking customer privacy. This approach is particularly valuable when testing marketing strategies, simulating diverse customer scenarios, or training AI models where data privacy is paramount.
To incorporate this, start with a small, anonymized sample of real data, then use algorithms to generate expanded datasets that maintain the statistical properties of the original. - Fills in data gaps: When your first-party data has limitations, such as underrepresented segments or limited historical information, synthetic data can fill these gaps, providing a more complete picture for your analytics and modeling efforts.
The Limitations
While both synthetic data and first-party data offer compelling advantages for your marketing, it's important to understand their limitations before building your strategy around them.
1st Party Data Limitations
First-party data is incredibly valuable because it comes directly from your audience, but it has several significant limitations:
Scaling challenges: Your first-party data is inherently limited to your existing customer base and those who have directly interacted with your brand. This creates a natural ceiling on how much data you can collect, making it difficult to scale your insights to broader audiences.
Data freshness and decay: Behavioral signals captured through first-party data can become outdated quickly, especially in fast-moving consumer categories. Someone who clicked on a product page two months ago may no longer be in-market, making it harder to act on stale data. This lag can be a major drawback for performance marketers who rely on real-time signals for targeting, personalization, and bid optimization.
System fragmentation: Most businesses store first-party data across multiple systems, such as CRMs, email platforms, analytics tools, and e-commerce platforms. This fragmentation makes it difficult to build a unified view of your customers. To overcome this, invest in data integration tools like a unified analytics dashboard that syncs information across platforms and creates a single customer view.
Regulatory constraints: With the rise of privacy regulations like GDPR and CCPA, collecting first-party data has become more restricted.
Synthetic Data Limitations
Synthetic data presents an innovative alternative, but it comes with its own set of limitations:
Accuracy concerns: Synthetic data is only as good as the models that create it. If not properly designed, it can miss important nuances in consumer behavior, leading to inaccurate predictions and strategies.
Inherent bias risks: If the original data used to generate synthetic datasets contains biases, these will likely be amplified in the synthetic output. Research found that synthetic datasets can introduce distortions that don't accurately reflect the characteristics and preferences of your target audience. For example, if your training data overrepresents high-intent shoppers who convert quickly, the resulting synthetic dataset might skew toward aggressive buyer behavior. This could lead your team to over-optimize campaigns for bottom-of-funnel conversions while neglecting awareness or consideration stage prospects.
Validation requirements: Synthetic data requires rigorous validation to maintain the statistical properties and relationships in the original data. This validation process can be resource-intensive and requires specialized expertise to get right. To validate properly, compare major statistical measures between your synthetic and real datasets, and test marketing models on both for similar outcomes.
Considering these limitations doesn't mean you should avoid using these data types. Rather, it helps you approach them with appropriate expectations and develop strategies to mitigate their weaknesses. The most potent approach often combines both data types while implementing proper validation processes for accuracy.
When Should You Use Synthetic Data vs. First-Party Data?
Choosing between synthetic and first-party data can impact your marketing success. Here's a scenario-by-scenario breakdown to help you decide when to use each:
Segmentation & Personalization → 1st Party Data
For understanding your actual customers and personalizing their experiences, first-party data remains the gold standard for developing personalized marketing strategies:
- Accuracy: Reflects real customer preferences and behaviors.
- Trust: Customers have directly shared this information with you.
- Depth: Captures nuanced emotional context that synthetic data might miss.
- Relevance: Directly represents your specific audience.
First-party data is irreplaceable when creating targeted campaigns, building customer segments, or designing personalized experiences.
Triggered Automations → 1st Party Data
First-party data is the better choice for creating responsive, automated marketing workflows for the following reasons:
- Reliability: Based on actual customer actions rather than simulations.
- Real-time relevance: Responds to genuine customer behaviors.
- Timing sensitivity: Captures the critical moments when customers are most receptive, allowing for helpful ad scheduling strategies.
First-party data helps your automated marketing respond appropriately to customer needs and behaviors.
A/B Testing & Model Training → Synthetic Data
Synthetic data offers several advantages when you need to test different approaches or train machine learning models.
- Privacy protection: You can conduct extensive testing without risking customer data exposure.
- Scale: Generate unlimited data points to ensure statistical significance.
- Edge cases: Create scenarios that might be rare in your actual customer base.
- Speed: Accelerate development cycles without waiting for real data collection.
This approach is particularly valuable when testing new features, simulating user journeys, or training AI models where data privacy is paramount. For instance, researchers at MIT recently showed that image classification models trained solely on high-quality synthetic data outperformed those trained on real-world images, proving synthetic data’s potential to reduce costs and boost training efficacy without sacrificing performance.
Simulating New Product Journeys → Synthetic Data
Synthetic data can provide valuable insights when launching new products or entering unfamiliar markets.
- Forecasting: Model potential customer behaviors without historical data.
- Risk Reduction: Test concepts in simulated environments before real-world launch.
- Scenario Planning: Prepare for various market responses.
Synthetic data allows you to explore hypothetical situations and prepare strategies before committing resources.
Decision Checklist
When determining which data type to use, ask yourself:
- Do you need detailed insights about your existing customers? → 1st Party Data
- Are you responding to specific customer actions? → 1st Party Data
- Is customer trust and relationship building your focus? → 1st Party Data
- Is privacy your primary concern? → Synthetic Data
- Are you exploring hypothetical scenarios? → Synthetic Data
- Do you need massive scale quickly? → Synthetic Data
The most sophisticated marketing strategies often integrate both types, using synthetic data to expand possibilities while maintaining first-party data as the foundation for customer understanding.
Why the Best Marketers Use Both Synthetic Data and First-Party Data
The most productive marketing strategies leverage first-party and synthetic data in complementary ways, creating a powerful synergy that exceeds what either data type could achieve alone.
When you integrate synthetic data with your first-party information, you immediately improve your analytical capabilities. First-party data provides the authentic foundation of actual customer behaviors and preferences, while synthetic data fills gaps, extends limited datasets, and allows for deeper analysis without privacy concerns.
The advantages of this combined approach are substantial:
Better segmentation: First-party data identifies your actual customer segments, while synthetic data helps model underrepresented groups and predict behaviors where your data is thin. Start by analyzing your first-party data to identify where you have knowledge gaps, then use synthetic data techniques to model those missing pieces.
Smarter testing: Using synthetic data lets you simulate various scenarios without risking real customer relationships. You can test messaging, offers, and experiences across synthesized audiences before committing real marketing dollars.
Privacy-compliant analytics: As privacy regulations tighten, synthetic data helps maintain analytical capabilities without risking compliance issues.
Scale and innovation: Synthetic data lets you scale beyond the limitations of your first-party data collection, permitting faster innovation and more extensive experimentation through AI in marketing automation.
To get the most out of both types of data, you need the right tools. Pixis offers no-code AI solutions that make it easy to use your data to improve targeting, automate creatives, and boost performance. Strategically combining both data types helps you make more informed decisions, develop more effective personalization strategies, and drive stronger marketing performance.
How Top Brands Use Both Data Types
Leading brands are discovering powerful ways to combine synthetic and first-party data for exceptional marketing results. Several examples include:
Amazon: Smarter Targeting with Real + Simulated Shopper Data
As third-party cookies decline, Amazon has leaned heavily into its rich reservoir of first-party data to power targeted advertising and recommendation engines. Through its Demand-Side Platform (DSP), Amazon allows advertisers to access behavioral insights derived from customer shopping activity, search history, and purchase behavior. This helps brands serve highly relevant ads based on real, in-the-moment user intent.
Amazon also experiments with synthetic data, modeling how hypothetical customers might behave when introduced to new product categories or geographies. This helps optimize campaigns quickly.
With the combination of real-world behavioral data and synthetic modeling, Amazon can fine-tune its personalization systems and improve conversion rates across its ecosystem.
Tesla: Building Better AI with Synthetic Driving Data
Tesla’s approach to synthetic data is rooted in its mission to advance autonomous driving technology. The company collects massive amounts of real-world driving data from its fleet. Still, this data can be sparse for rare edge cases (like unexpected pedestrian movements or unusual weather conditions).
To address this, Tesla generates synthetic datasets by altering real sensor input or creating fully simulated driving environments. These synthetic scenarios allow Tesla to train its self-driving algorithms more effectively without waiting for those rare events to occur in the real world.
This practice is then paired with first-party vehicle telemetry and user interaction data (such as in-app behavior or charging habits), which helps Tesla refine both its AI systems and its customer-facing feature rollouts.
Final Thoughts
In a world where data privacy and personalization are high priorities, knowing how to use both first-party and synthetic data productively is vital for B2C marketers. First-party data offers unmatched accuracy and trust; it’s a reliable source for understanding customer behavior and delivering personalized, regulation-compliant experiences. Synthetic data, on the other hand, unlocks speed, scale, and safety, making it a powerful tool for testing, AI training, and scenario modeling without risking privacy.
Each data type has unique strengths. The important part is knowing when and how to use each to support your broader marketing goals.